Cleaning Up Dirty Data: How Inaccurate Data Creates Member Distrust (and How to Fix it)
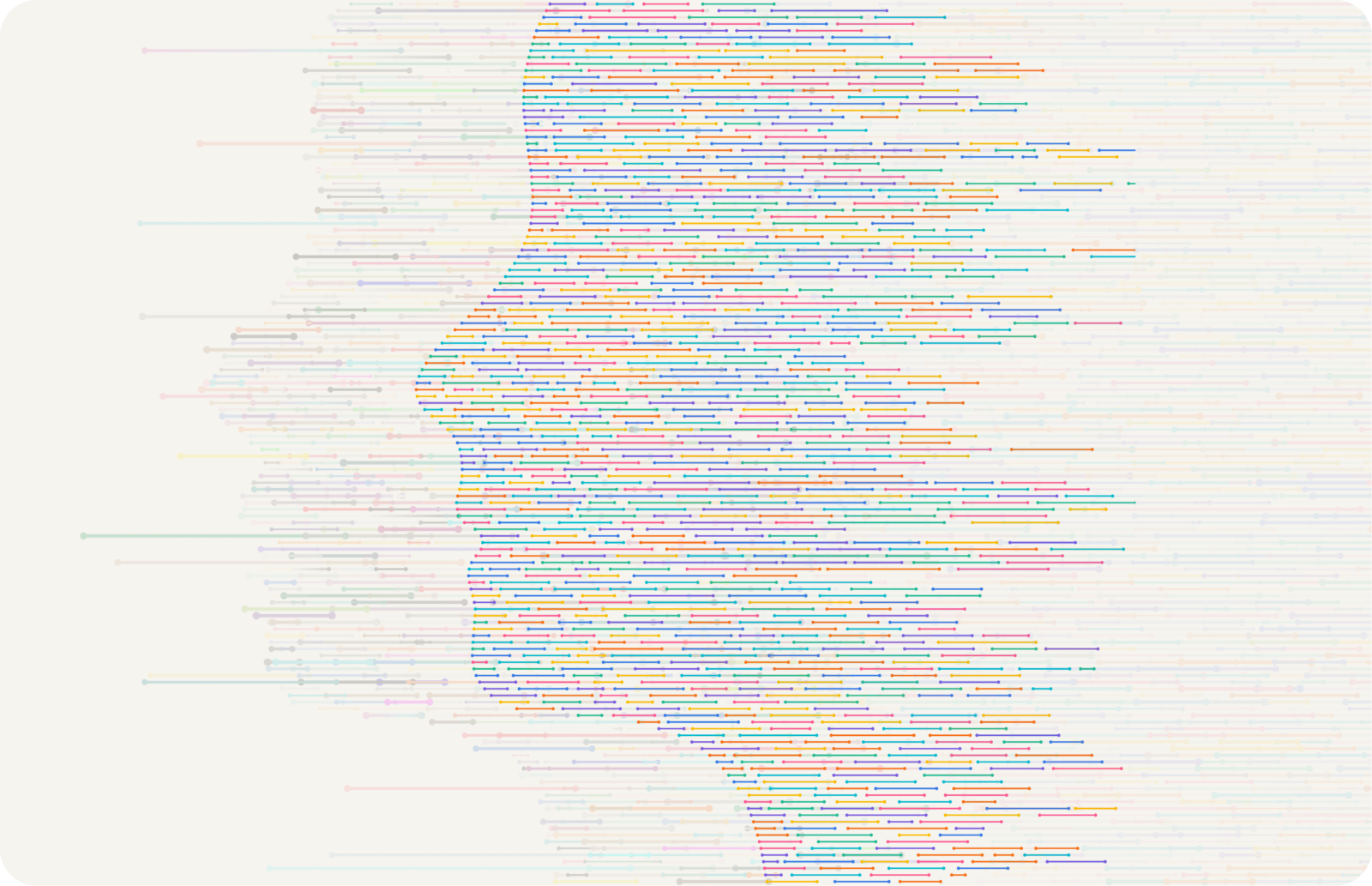
Data can be an excellent tool—or a liability—when it comes to understanding members and building trust. At its best, member data can help providers understand a member’s needs and get a clearer picture of their overall health. However, “dirty data,” (data that is incomplete or inaccurate) leads to missteps that create distrust amongst members who have been underserved and underrepresented within health care systems.
Curt Theis, Reema’s Head of Data, shared his insights regarding data’s role in health care and how it can impact members.
How does “dirty data” get created within a health care system?
When we think about data within the healthcare system, a lot of information comes from claims that are submitted to health insurance plans by providers. For the most part, the data on a claim that is required for it to be processed will be fairly accurate, in terms of the services or procedures provided, and any drugs that were administered.
Sometimes information like diagnosis codes are not required for the claim to be processed, so that information may be missing or inaccurate, which prevents us from having a full picture of the member’s health. Another factor that shapes the accuracy of the data we have is that claims typically reflect when treatment was provided, so we don’t have information about the outcomes of the treatment.
As a result, the data health plans have is imperfect at best. Ultimately, when it is shared with us, we’re trying to piece together the bigger picture of a member’s health from fragmented pieces of information.
How does data impact member experiences?
The issue of member contact information is a great example. This type of data can be challenging for health plans to maintain in a centralized way. However, if a member’s contact information is incomplete, or incorrect, it can determine whether or not we’re able to reach them and help connect them to care and services they may need.
On another level, if a provider has inaccurate information about a member’s diagnosis, it’s difficult for them to develop an understanding of what the member’s health issues might be. For example, if a diagnosis code incorrectly indicates that a member currently has a heart condition when they have a history of cardiac issues, this will make a significant difference in the recommendations a provider makes regarding the member’s perceived health needs.
When we are trying to engage members who have been underserved by health care systems, having an accurate understanding of their situation is critical to establishing trust.
When inaccuracies like this occur, the impact is that the member may feel skeptical about whether their provider has a baseline understanding of their current health.
From your perspective, what is one way that data collection could be improved?
One way to think about cleaning up data would be for health plans to check against medical records so that they can understand the discrepancies that might appear between a member’s medical record and a claim. These discrepancies can create misperceptions about illness or diagnoses that can have implications at the population level.
For example, there is a lot of information about mental health that assumes we know approximately how many people have depression in our population. I conducted a study where I compared the most specific definition of depression, which is that somebody has a diagnosis and is taking medication, against populations that just have a diagnosis, or are just taking antidepressants. What I found is that, the most specific definition indicated that less than 2% of the population would be considered as having depression, whereas people with just a diagnosis represented 3%, and people taking antidepressants were 15%.
A key takeaway from the study is that if we’re trying to understand how many people have depression, we have to think carefully about what definition we use, and which one will help us correctly identify what percentage of the population has depression. The differences between those numbers are significant, and they illustrate what can be at stake when we’re gathering and interpreting data.
How is Reema working to help ensure that member data is accurate?
At the ground level, we know that our Community Guides may have inaccurate or incomplete data, so we’ve developed processes for our Guides to try to understand what our members are experiencing. They can ensure that this information is logged accurately in our system so that it can be referenced internally as we work with that member.
We also work with health plans directly to navigate the complexities of sharing member data with us. This can range from understanding the privacy concerns around sharing particular types of data, to helping them to figure out the backend systems needed to access the data, and being in dialogue with their legal team about our privacy coverage and capabilities.